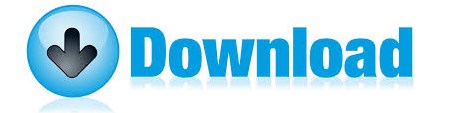
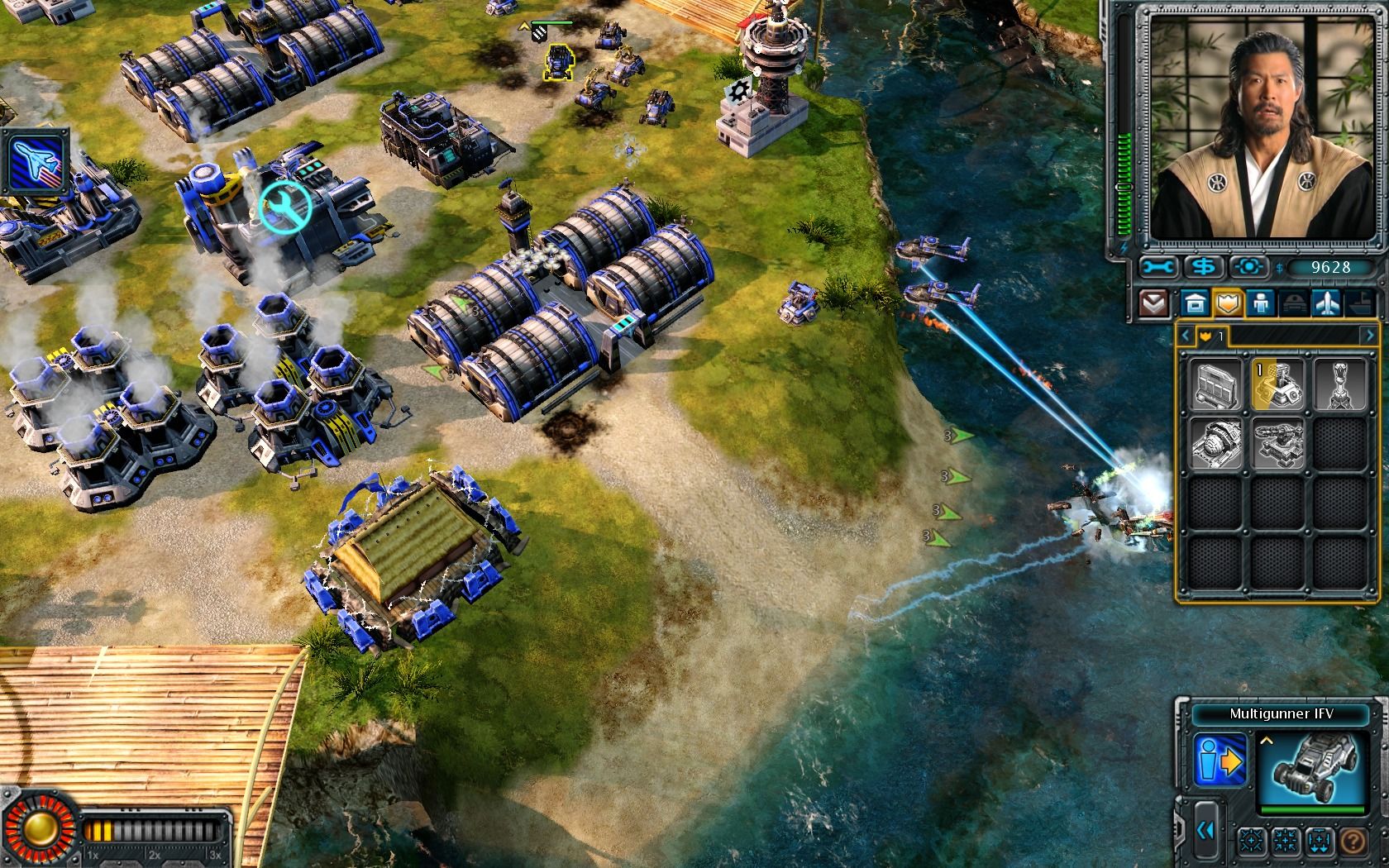
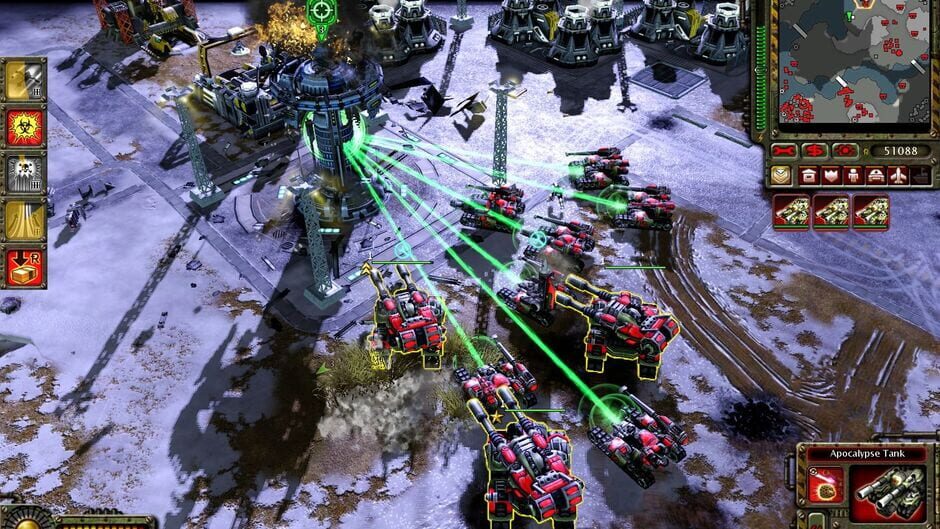
This entails active monitoring and proactive mechanisms to prevent their manifestation and mutual effects. Put differently, we cannot appropriately prepare for or mitigate any of these risks unless due attention is paid to all of them. While prior work has argued for treating each type of risk seriously and urgently, these risks - however unlikely or difficult to imagine today - are likely to reverberate and exacerbate each other unless we properly address and mitigate them. Although many real-world examples of risks may fit into more than one of those categories, we emphasize these categories for their crucial analytical distinctions and independent importance for ensuring that the future development of AI systems remains safe and commensurate with human priorities. We focus on three broad categories of risks: to democracy and security, to human rights and well-being, and of global catastrophes. By "sociotechnical," we refer to the variety of interfaces between emerging AI systems and the legacy systems (political, cultural, cognitive, economic) with which formal AI models will interact.
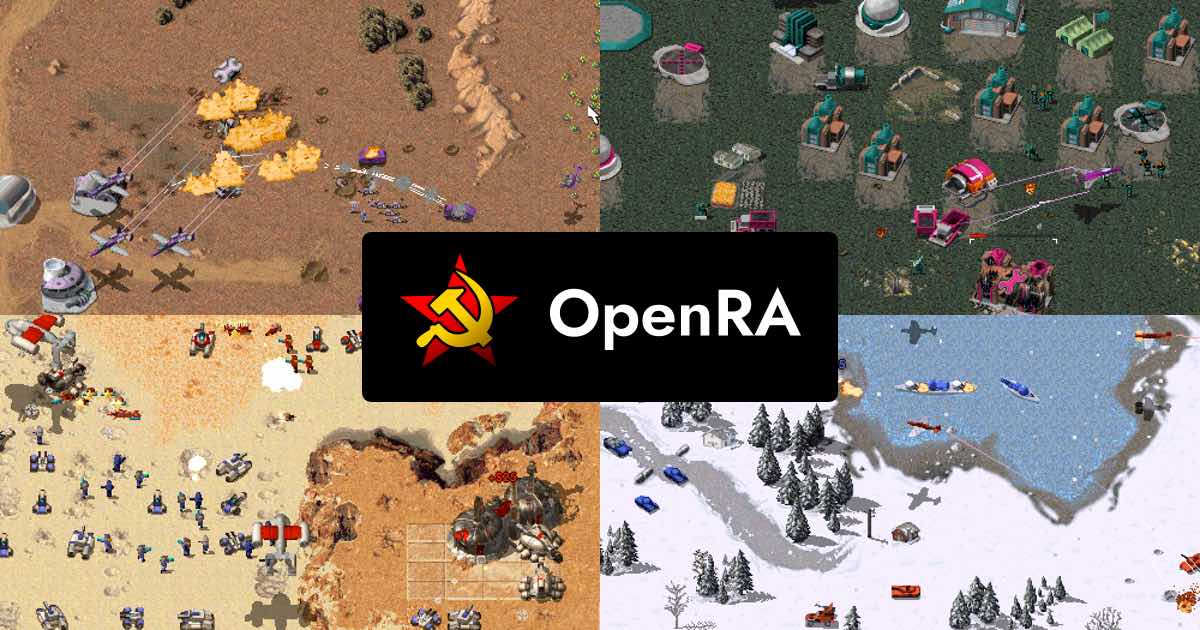
In our submission to NIST, we highlight the irreducibly sociotechnical contexts in which risks are likely to manifest and be experienced by stakeholders. The AI RMF should integrate a diversity of lenses on AI risks, from the potential worsening of inequalities, to the pressing gaps in meaningful transparency and explainability, to the harms that may emerge from formal (mathematical/statistical) models that have been misspecified with respect to human values and goals. The AI RMF is an important opportunity to develop risk assessment and mitigation measures that can help prevent the harms people are experiencing from AI systems today and prevent potential future harms from materializing. Much as automobiles, airplanes, and the internet once seemed far-fetched, even as their technical capabilities were within reach, these applications will become so commonplace and thoroughly integrated into daily behavior that it will be difficult to imagine social life without them. Many AI systems with controversial risks are already in use, including cars with self-driving capabilities, automated decision systems, and human-level language models.
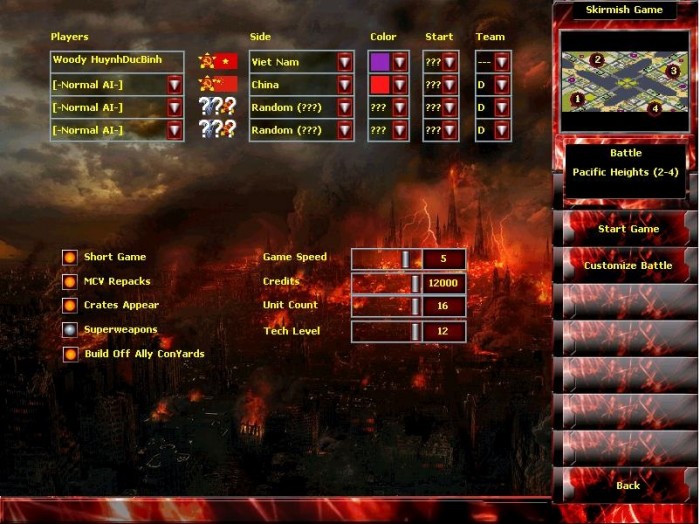
Inconsistent terminology, uncertainty about future developments, and disciplinary divisions limit our capacity to make sense of the risks posed by AI today, and to accurately predict and prepare for future risks. There are significant challenges associated with developing an effective AI risk management framework. We provided a comment to NIST, which we frame and summarize below.
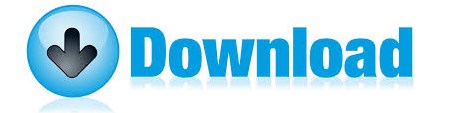